Tricia Phillips, senior vice president of product and strategy at Kount, Inc., advocates for machine learning and biometrics in securing business data and information.
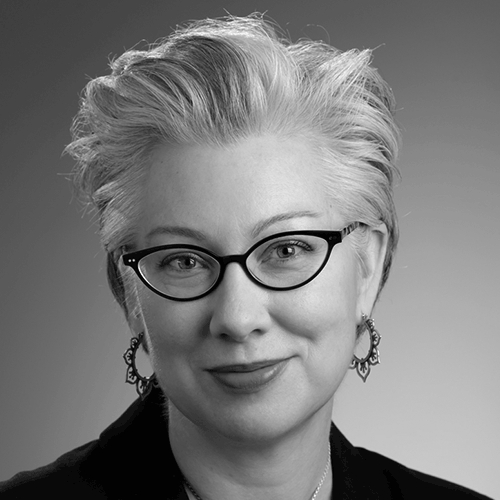
Tricia Phillips
Senior Vice President of Product and Strategy, Kount Inc.
What is the biggest mistake that companies make when it comes to data and information security?
The biggest mistake B2C companies make from a cybersecurity standpoint is viewing the risk of fraud or account compromise in a vacuum, without considering the business risk of a poor customer experience and lost revenue. Protecting customer data and the business against fraud cannot come at the cost of the customer experience and conversion. Both are critically important from a brand and revenue standpoint, and should be balanced based on the reasonable risk of business impact.
What should businesses be looking for in a fraud and risk management solution provider? What questions should they be asking to make sure their anti-fraud solutions vendor is the right fit for them?
Today’s digital businesses need a blend of modern technology and human expertise. There are no predictive machine learning models which can prevent fraud with low false positives without the aid of humans. It takes people with a strong understanding of the specific use case to define the machine learning features, train the models and continually reinforce and supervise the models. A comprehensive fraud prevention solution must include machine learning (preferably both supervised and unsupervised), an engine supporting business policies specific to product types, regions and other factors, an investigation/analysis capability and the ability to evaluate outcome data to enable continuous improvement and evolution.
How is machine learning being used to better prevent fraud?
Machine learning can be used by a fraud team to identify new fraud trends and to better identify low-risk activity, to ensure that good customers are inconvenienced as little as possible in the fight against fraud.
Relatively simple, supervised machine learning has been used in fraud detection and underwriting for decades. The difference now is that the enhancements in computing and data processing technologies have enabled the productionalization of advanced data analytics tools and theories (which used to exist almost exclusively in academia) to work in real time to detect anomalies and calculate the likelihood of fraud.
Unsupervised machine learning is used for anomaly detection — to catch emerging fraud trends which haven’t been explicitly observed or modeled before. Supervised machine learning tends to be used as a predictive method of detecting and preventing fraud, and is based on historical behaviors and outcomes. It used to be that a supervised machine learning model would be developed on data from 3-12 months prior, and would take six months to analyze and build. By the time these models were released into production, they were already over nine months behind the latest fraud trends. Today, the tools exist to update models much more quickly for predictive value.
What is the biggest advantage to having biometric security solutions when protecting businesses from fraud?
The allure of biometrics as an authentication method is the idea that, theoretically, they can’t be compromised. The reality is that most biometric authentication methods can still be compromised by a determined attacker because liveness detection (or presentation attack detection) is still underdeveloped in the hardware technologies available in most B2C authentication scenarios. Are biometrics harder to break than passwords? Yes, usually they are, but in most cases, they are not without points of failure.
What do businesses need to consider before introducing biometrics?
Consider biometrics (whether physical or behavioral) to be a means of reducing friction in an authentication scenario by enabling further corroboration of the identity of a known customer when combined with other familiarity signals.